Contents
What’s New✨
Inviting for Seminars
I am organizing the 2025 fall Seminar Series in Statistics at Guanghua School of Management, Peking University. If you are traveling to Beijing in the fall, please let me know. Proposals are welcome!
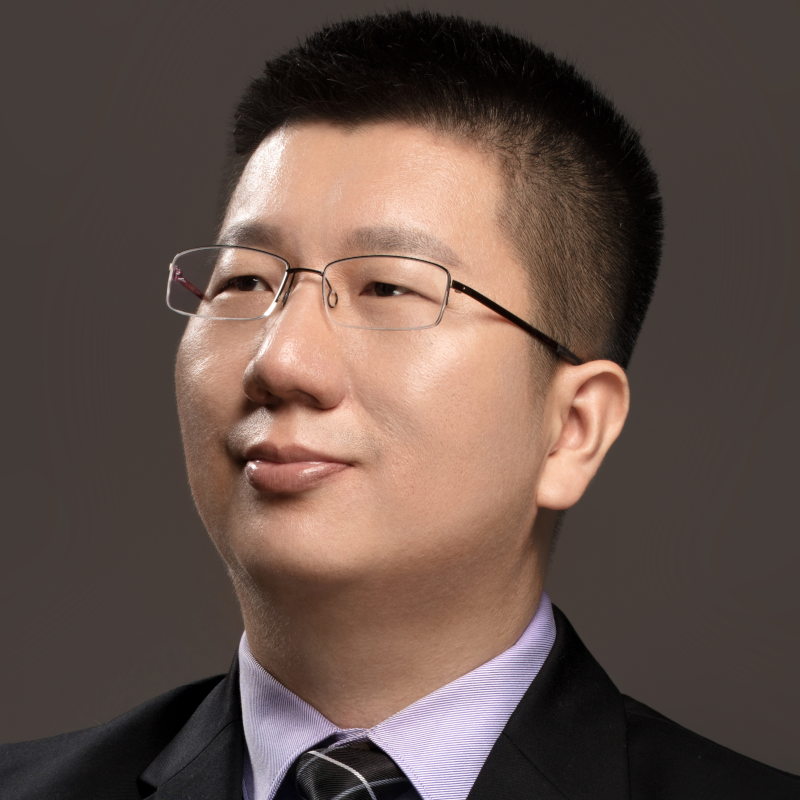
Dr. Feng Li (ORCiD: 0000-0002-4248-9778) joined the Guanghua School of Management at Peking University in 2024 as an Associate Professor in the Department of Business Statistics and Econometrics. Prior to that, he was an Associate Professor of Statistics at Central University of Finance and Economics in Beijing, China, where he also served as the Associate Dean for the School of Statistics and Mathematics from 2016 to 2022. He earned his Ph.D. in Statistics from Stockholm University, Sweden in 2013.
Dr. Feng Li’s research interests include Bayesian Statistics, Econometrics and Forecasting, and Distributed Learning. He develops highly scalable algorithms and software for solving real business problems. His recent research output appeared in field top-tier journals like European Journal of Operational Research (ABS4), Contemporary Accounting Research (FT50), Journal of Business and Economic Statistics (ABS4), Journal of Computational and Graphical Statistics, International Journal of Forecasting. Feng Li has presented at the world meeting of the International Society for Bayesian Analysis (ISBA), and International Symposium on Forecasting.
📢📢📢 Dr. Feng Li is seeking motivated PhD candidates in Statistics to join the team. The ideal candidates will have a strong foundation in statistical theory and computational skills. Successful candidates will engage in cutting-edge research, benefit from an interdisciplinary environment, and contribute to advancements in both statistics and related fields.
Education 🎓
- Ph.D., 2013, Statistics, Stockholm University, Sweden.
- Thesis: Bayesian Modeling of Conditional Densities (The 2014 Cramér Prize for the best Ph.D. thesis in Statistics and Mathematical Statistics in Sweden)
- Supervisor: Prof. Mattias Villani
- Thesis opponent: Prof. Sylvia Frühwirth-Schnatter
- M.S., 2008, Statistics, Dalarna University, Sweden.
- B.S., 2007, Statistics, Renmin University of China (Graduated with Outstanding Award, honored by Beijing Municipal Education Commission)
Employment History
- 2013 September – 2024 June, Assitant Professor and Associate Professor, School of Statistics and Mathematics, Central University of Finance and Economics, Beijing 102206, China
- 2016 July – 2022 December, Associate Dean, School of Statistics and Mathematics, Central University of Finance and Economics, Beijing 102206, China
Teaching
Dr. Feng Li is offering two courses Big Data Computation and Forecasting and Forecasting with AI at Guanghua School of Management, Peking University.
Let’s fika! ☕
Every Monday 13:00 to 14:00 during the semester, Dr. Feng Li invites students to join him in the office for a delightful coffee break (fika in Swedish). Students are welcome to discuss research ideas and share experiences about their studies and personal growth!
Office: | Room 420, Guanghua Building 2 No. 5 Yiheyuan Road, Haidian District, Beijing 100871, China |
Email: | feng.li@gsm.pku.edu.cn |
Tel. | +86 (010) 6274 7602 |
CV: | Curriculum Vitae 中文简历 |
Grants ⚙️
- Statistical Learning and Management Practices in Large-Scale Business Scenarios. Funded by National Natural Science Foundation of China (2025+). Major Investigator (CNY 500,000).
- Evaluation on Sports Betting Market. Funded by the Hong Kong Jockey Club (Beijing) (2024+). Principal Investigator. (CNY 670,000)
- Hierarchical economic forecasting from a global modelling perspective. Funded by the National Social Science Fund of China (2022- ). Principal Investigator (CNY 200,000).
- Complex Time Series Forecasting for E-commerce. Funded by Alibaba Innovative Research Program (2021-2023 ). Principal investigator (CNY 480,000).
- Development of the Methodologies of Objective Performance Criteria Based Single-Armed Trials for The Clinical Evaluation of Traditional Chinese Medicine. Funded by the National Natural Science Foundation of China (2020- ). Major Investigator (CNY 150,000).
- Efficient Bayesian Flexible Density Methods with High Dimensional Financial Data funded by the National Natural Science Foundation of China (2016-2019). Principal investigator (CNY 200,000).
- Bayesian Multivariate Density Estimation Methods for Complex Data. Funded by the Ministry of Education, China (2014-2016). Principal investigator (CNY 50,000).
Working Papers⏳
- Xiaoqian Wang, Yanfei Kang, and Feng Li (2022). Another look at forecast trimming for combinations: robustness, accuracy and diversity. Working paper
Publications🗞️
-
Abstract: 本文对结构性变化的旅游需求进行研究,基于带有外生变量的向量自回归(VARX)模型,提出了一种分段组合预测的方法。与既有研究普遍采用的基于完整数据集构建组合预测模型不同,本文创新性地将时间因素纳入组合预测考量,通过将不同时间段的变量视为独立的单元,构建出分段时间序列数据集的组合预测模型。该方法以游客的网络搜索行为作为外生变量用于预测旅游人数,并捕捉这些外生变量在不同时间节点上对旅游人数产生的差异化影响,特别是在新冠疫情等突发冲击下的动态变化。实证结果显示,VARX模型的分段组合在预测中国出境旅游人数时展现出更高的准确性,其预测精度因考虑了外生变量在不同时间段的特异性影响而得以提升。事后分析进一步显示,特别是针对2024年中国出境旅游趋势的外样本预测结果,随着新冠疫情影响的逐渐消退及全球旅游市场的逐步复苏,中国出境旅游人数将呈现积极向上的增长态势。这一结论与现有公开文献中的趋势分析相吻合,进一步印证了本文预测方法的实践应用价值。BibTeX:
@article{WangWen2025VARX, author = {王雯 and 李丰}, title = {基于分段组合VARX模型的中国出境游客数量预测}, journal = {经济管理学刊}, year = {2025}, volume = {4}, pages = {255--284}, doi = {10.20180/j.qjem.2025.01.09} }
-
Yiming Zhong, Yinuo Ren, Guangyao Cao, Feng Li and Haobo Qi (2025). “Optimal Starting Point for Time Series Forecasting”. Expert Systems with Applications, Vol. 273pp. 126798.Abstract: Recent advances on time series forecasting mainly focus on improving the forecasting models themselves. However, when the time series data suffer from potential structural breaks or concept drifts, the forecasting performance might be significantly reduced. In this paper, we introduce a novel approach called Optimal Starting Point Time Series Forecast (OSP-TSP) for optimal forecasting, which can be combined with existing time series forecasting models. By adjusting the sequence length via leveraging the XGBoost and LightGBM models, the proposed approach can determine the optimal starting point (OSP) of the time series and then enhance the prediction performances of the base forecasting models. To illustrate the effectiveness of the proposed approach, comprehensive empirical analysis have been conducted on the M4 dataset and other real world datasets. Empirical results indicate that predictions based on the OSP-TSP approach consistently outperform those using the complete time series dataset. Moreover, comparison results reveals that combining our approach with existing forecasting models can achieve better prediction accuracy, which also reflect the advantages of the proposed approach.BibTeX:
@article{ZhongY2025OptimalStarting, author = {Zhong, Yiming and Ren, Yinuo and Cao, Guangyao and Li, Feng and Qi, Haobo}, title = {Optimal Starting Point for Time Series Forecasting}, journal = {Expert Systems with Applications}, year = {2025}, volume = {273}, pages = {126798}, url = {https://www.sciencedirect.com/science/article/pii/S0957417425004208}, doi = {10.1016/j.eswa.2025.126798} }
-
Han Wang, Wen Wang, Feng Li, Yanfei Kang and Han Li (2025). “Catastrophe Duration and Loss Prediction via Natural Language Processing”. Variance, Vol. 18Abstract: Textual information from online news is more timely than insurance claim data during catastrophes, and there is value in using this information to achieve earlier damage estimates. In this paper, we use text-based information to predict the duration and severity of catastrophes. We construct text vectors through Word2Vec and BERT models, using Random Forest, LightGBM, and XGBoost as different learners, all of which show more satisfactory prediction results. This new approach is informative in providing timely warnings of the severity of a catastrophe, which can aid decision-making and support appropriate responses.BibTeX:
@article{WangH2024CatastropheDuration, author = {Wang, Han and Wang, Wen and Li, Feng and Kang, Yanfei and Li, Han}, title = {Catastrophe Duration and Loss Prediction via Natural Language Processing}, journal = {Variance}, year = {2025}, volume = {18}, url = {https://variancejournal.org/article/133589-catastrophe-duration-and-loss-prediction-via-natural-language-processing} }
-
Yuqin Huang, Feng Li, Tong Li and Tse-Chun Lin (2024). “Local Information Advantage and Stock Returns: Evidence from Social Media”. Contemporary Accounting Research, Vol. 41(2), pp. 1089-1119.Abstract: We examine the information asymmetry between local and nonlocal investors with a large dataset of stock message board postings. We document that abnormal relative postings of a firm, i.e., unusual changes in the volume of postings from local versus nonlocal investors, capture locals’ information advantage. This measure positively predicts firms’ short-term stock returns as well as those of peer firms in the same city. Sentiment analysis shows that posting activities primarily reflect good news, potentially due to social transmission bias and short-sales constraints. We identify the information driving return predictability through content-based analysis. Abnormal relative postings also lead analysts’ forecast revisions. Overall, investors’ interactions on social media contain valuable geography-based private information.BibTeX:
@article{HuangY2024LocalInformation, author = {Huang, Yuqin and Li, Feng and Li, Tong and Lin, Tse-Chun}, title = {Local Information Advantage and Stock Returns: Evidence from Social Media}, journal = {Contemporary Accounting Research}, year = {2024}, volume = {41}, number = {2}, pages = {1089--1119}, url = {http://doi.org/10.2139/ssrn.2501937}, doi = {10.1111/1911-3846.12935} }
-
Yuan Gao, Rui Pan, Feng Li, Riquan Zhang and Hansheng Wang (2024). “Grid Point Approximation for Distributed Nonparametric Smoothing and Prediction”. Journal of Computational and Graphical Statistics, pp. 1-29.Abstract: Kernel smoothing is a widely used nonparametric method in modern statistical analysis. The problem of efficiently conducting kernel smoothing for a massive dataset on a distributed system is a problem of great importance. In this work, we find that the popularly used one-shot type estimator is highly inefficient for prediction purposes. To this end, we propose a novel grid point approximation (GPA) method, which has the following advantages. First, the resulting GPA estimator is as statistically efficient as the global estimator under mild conditions. Second, it requires no communication and is extremely efficient in terms of computation for prediction. Third, it is applicable to the case where the data are not randomly distributed across different machines. To select a suitable bandwidth, two novel bandwidth selectors are further developed and theoretically supported. Extensive numerical studies are conducted to corroborate our theoretical findings. Two real data examples are also provided to demonstrate the usefulness of our GPA method.BibTeX:
@article{GaoY2024GridPoint, author = {Gao, Yuan and Pan, Rui and Li, Feng and Zhang, Riquan and Wang, Hansheng}, title = {Grid Point Approximation for Distributed Nonparametric Smoothing and Prediction}, journal = {Journal of Computational and Graphical Statistics}, year = {2024}, pages = {1--29}, url = {https://arxiv.org/abs/2409.14079}, doi = {10.1080/10618600.2024.2409817} }
-
Feng Li (2024). “Book Review of Causality: Models, Reasoning, and Inference, Judea Pearl. (Second Edition). (2009)”. International Journal of Forecasting, Vol. 40(1), pp. 423-425.Abstract: With the big popularity and success of Judea Pearl’s original causality book, this review covers the main topics updated in the second edition in 2009 and illustrates an easy-to-follow causal inference strategy in a forecast scenario. It further discusses some potential benefits and challenges for causal inference with time series forecasting when modeling the counterfactuals, estimating the uncertainty and incorporating prior knowledge to estimate causal effects in different forecasting scenarios.BibTeX:
@article{LiF2024ForecasterReview, author = {Li, Feng}, title = {Book Review of Causality: Models, Reasoning, and Inference, Judea Pearl. (Second Edition). (2009)}, journal = {International Journal of Forecasting}, year = {2024}, volume = {40}, number = {1}, pages = {423--425}, url = {http://arxiv.org/abs/2308.05451}, doi = {10.1016/j.ijforecast.2023.08.005} }
-
Guanyu Zhang, Feng Li and Yanfei Kang (2023). “Probabilistic Forecast Reconciliation with Kullback-Leibler Divergence Regularization”. In 2023 IEEE International Conference on Data Mining Workshops (ICDMW). pp. 601-607.Abstract: As the popularity of hierarchical point forecast reconciliation methods increases, there is a growing interest in probabilistic forecast reconciliation. Many studies have utilized machine learning or deep learning techniques to implement probabilistic forecasting reconciliation and have made notable progress. However, these methods treat the reconciliation step as a fixed and hard post-processing step, leading to a trade-off between accuracy and coherency. In this paper, we propose a new approach for probabilistic forecast reconciliation. Unlike existing approaches, our proposed approach fuses the prediction step and reconciliation step into a deep learning framework, making the reconciliation step more flexible and soft by introducing the Kullback-Leibler divergence regularization term into the loss function. The approach is evaluated using three hierarchical time series datasets, which shows the advantages of our approach over other probabilistic forecast reconciliation methods.BibTeX:
@inproceedings{ZhangG2023ProbabilisticForecast, author = {Zhang, Guanyu and Li, Feng and Kang, Yanfei}, title = {Probabilistic Forecast Reconciliation with Kullback-Leibler Divergence Regularization}, booktitle = {2023 IEEE International Conference on Data Mining Workshops (ICDMW)}, year = {2023}, pages = {601--607}, url = {https://arxiv.org/abs/2311.12279}, doi = {10.1109/ICDMW60847.2023.00084} }
-
Yinuo Ren, Feng Li, Yanfei Kang and Jue Wang (2023). “Infinite Forecast Combinations Based on Dirichlet Process”. In 2023 IEEE International Conference on Data Mining Workshops (ICDMW). pp. 579-587.Abstract: Forecast combination integrates information from various sources by consolidating multiple forecast results from the target time series. Instead of the need to select a single optimal forecasting model, this paper introduces a deep learning ensemble forecasting model based on the Dirichlet process. Initially, the learning rate is sampled with three basis distributions as hyperparameters to convert the infinite mixture into a finite one. All checkpoints are collected to establish a deep learning sub-model pool, and weight adjustment and diversity strategies are developed during the combination process. The main advantage of this method is its ability to generate the required base learners through a single training process, utilizing the decaying strategy to tackle the challenge posed by the stochastic nature of gradient descent in determining the optimal learning rate. To ensure the method’s generalizability and competitiveness, this paper conducts an empirical analysis using the weekly dataset from the M4 competition and explores sensitivity to the number of models to be combined. The results demonstrate that the ensemble model proposed offers substantial improvements in prediction accuracy and stability compared to a single benchmark model.BibTeX:
@inproceedings{RenY2023InfiniteForecast, author = {Ren, Yinuo and Li, Feng and Kang, Yanfei and Wang, Jue}, title = {Infinite Forecast Combinations Based on Dirichlet Process}, booktitle = {2023 IEEE International Conference on Data Mining Workshops (ICDMW)}, year = {2023}, pages = {579--587}, url = {https://arxiv.org/abs/2311.12379}, doi = {10.1109/ICDMW60847.2023.00081} }
-
Li Li, Yanfei Kang, Fotios Petropoulos and Feng Li (2023). “Feature-Based Intermittent Demand Forecast Combinations: Accuracy and Inventory Implications”. International Journal of Production Research, Vol. 61(22), pp. 7557-7572.Abstract: Intermittent demand forecasting is a ubiquitous and challenging problem in production systems and supply chain management. In recent years, there has been a growing focus on developing forecasting approaches for intermittent demand from academic and practical perspectives. However, limited attention has been given to forecast combination methods, which have achieved competitive performance in forecasting fast-moving time series. The current study aims to examine the empirical outcomes of some existing forecast combination methods and propose a generalized feature-based framework for intermittent demand forecasting. The proposed framework has been shown to improve the accuracy of point and quantile forecasts based on two real data sets. Further, some analysis of features, forecasting pools and computational efficiency is also provided. The findings indicate the intelligibility and flexibility of the proposed approach in intermittent demand forecasting and offer insights regarding inventory decisions.BibTeX:
@article{LiL2023FeaturebasedIntermittent, author = {Li, Li and Kang, Yanfei and Petropoulos, Fotios and Li, Feng}, title = {Feature-Based Intermittent Demand Forecast Combinations: Accuracy and Inventory Implications}, journal = {International Journal of Production Research}, year = {2023}, volume = {61}, number = {22}, pages = {7557--7572}, url = {https://arxiv.org/abs/2204.08283}, doi = {10.1080/00207543.2022.2153941} }
-
Xiaoqian Wang, Rob J. Hyndman, Feng Li and Yanfei Kang (2023). “Forecast Combinations: An over 50-Year Review”. International Journal of Forecasting, Vol. 39(4), pp. 1518-1547.Abstract: Forecast combinations have flourished remarkably in the forecasting community and, in recent years, have become part of mainstream forecasting research and activities. Combining multiple forecasts produced for a target time series is now widely used to improve accuracy through the integration of information gleaned from different sources, thereby avoiding the need to identify a single “best” forecast. Combination schemes have evolved from simple combination methods without estimation to sophisticated techniques involving time-varying weights, nonlinear combinations, correlations among components, and cross-learning. They include combining point forecasts and combining probabilistic forecasts. This paper provides an up-to-date review of the extensive literature on forecast combinations and a reference to available open-source software implementations. We discuss the potential and limitations of various methods and highlight how these ideas have developed over time. Some crucial issues concerning the utility of forecast combinations are also surveyed. Finally, we conclude with current research gaps and potential insights for future research.BibTeX:
@article{WangX2023ForecastCombinations, author = {Wang, Xiaoqian and Hyndman, Rob J. and Li, Feng and Kang, Yanfei}, title = {Forecast Combinations: An over 50-Year Review}, journal = {International Journal of Forecasting}, year = {2023}, volume = {39}, number = {4}, pages = {1518--1547}, url = {https://arxiv.org/abs/2205.04216}, doi = {10.1016/j.ijforecast.2022.11.005} }
-
Li Li, Yanfei Kang and Feng Li (2023). “Bayesian Forecast Combination Using Time-Varying Features”. International Journal of Forecasting, Vol. 39(3), pp. 1287-1302.Abstract: In this work, we propose a novel framework for density forecast combination by constructing time-varying weights based on time-varying features. Our framework estimates weights in the forecast combination via Bayesian log predictive scores, in which the optimal forecast combination is determined by time series features from historical information. In particular, we use an automatic Bayesian variable selection method to identify the importance of different features. To this end, our approach has better interpretability compared to other black-box forecasting combination schemes. We apply our framework to stock market data and M3 competition data. Based on our structure, a simple maximum-a-posteriori scheme outperforms benchmark methods, and Bayesian variable selection can further enhance the accuracy for both point forecasts and density forecasts.BibTeX:
@article{LiL2023BayesianForecast, author = {Li, Li and Kang, Yanfei and Li, Feng}, title = {Bayesian Forecast Combination Using Time-Varying Features}, journal = {International Journal of Forecasting}, year = {2023}, volume = {39}, number = {3}, pages = {1287--1302}, url = {https://arxiv.org/abs/2108.02082}, doi = {10.1016/j.ijforecast.2022.06.002} }
-
Xiaoqian Wang, Yanfei Kang, Rob J. Hyndman and Feng Li (2023). “Distributed ARIMA Models for Ultra-Long Time Series”. International Journal of Forecasting, Vol. 39(3), pp. 1163-1184.Abstract: Providing forecasts for ultra-long time series plays a vital role in various activities, such as investment decisions, industrial production arrangements, and farm management. This paper develops a novel distributed forecasting framework to tackle the challenges of forecasting ultra-long time series using the industry-standard MapReduce framework. The proposed model combination approach retains the local time dependency. It utilizes a straightforward splitting across samples to facilitate distributed forecasting by combining the local estimators of time series models delivered from worker nodes and minimizing a global loss function. Instead of unrealistically assuming the data generating process (DGP) of an ultra-long time series stays invariant, we only make assumptions on the DGP of subseries spanning shorter time periods. We investigate the performance of the proposed approach with AutoRegressive Integrated Moving Average (ARIMA) models using the real data application as well as numerical simulations. Our approach improves forecasting accuracy and computational efficiency in point forecasts and prediction intervals, especially for longer forecast horizons, compared to directly fitting the whole data with ARIMA models. Moreover, we explore some potential factors that may affect the forecasting performance of our approach.BibTeX:
@article{WangX2023DistributedARIMA, author = {Wang, Xiaoqian and Kang, Yanfei and Hyndman, Rob J. and Li, Feng}, title = {Distributed ARIMA Models for Ultra-Long Time Series}, journal = {International Journal of Forecasting}, year = {2023}, volume = {39}, number = {3}, pages = {1163--1184}, url = {https://arxiv.org/abs/2007.09577}, doi = {10.1016/j.ijforecast.2022.05.001} }
-
Bohan Zhang, Yanfei Kang, Anastasios Panagiotelis and Feng Li (2023). “Optimal Reconciliation with Immutable Forecasts”. European Journal of Operational Research, Vol. 308(1), pp. 650-660.Abstract: The practical importance of coherent forecasts in hierarchical forecasting has inspired many studies on forecast reconciliation. Under this approach, so-called base forecasts are produced for every series in the hierarchy and are subsequently adjusted to be coherent in a second reconciliation step. Reconciliation methods have been shown to improve forecast accuracy, but will, in general, adjust the base forecast of every series. However, in an operational context, it is sometimes necessary or beneficial to keep forecasts of some variables unchanged after forecast reconciliation. In this paper, we formulate reconciliation methodology that keeps forecasts of a pre-specified subset of variables unchanged or “immutable”. In contrast to existing approaches, these immutable forecasts need not all come from the same level of a hierarchy, and our method can also be applied to grouped hierarchies. We prove that our approach preserves unbiasedness in base forecasts. Our method can also account for correlations between base forecasting errors and ensure non-negativity of forecasts. We also perform empirical experiments, including an application to sales of a large scale online retailer, to assess the impacts of our proposed methodology.BibTeX:
@article{ZhangB2023OptimalReconciliation, author = {Zhang, Bohan and Kang, Yanfei and Panagiotelis, Anastasios and Li, Feng}, title = {Optimal Reconciliation with Immutable Forecasts}, journal = {European Journal of Operational Research}, year = {2023}, volume = {308}, number = {1}, pages = {650--660}, url = {http://arxiv.org/abs/2204.09231}, doi = {10.1016/j.ejor.2022.11.035} }
-
Li Li, Feng Li and Yanfei Kang (2023). “Forecasting Large Collections of Time Series: Feature-Based Methods”. In Forecasting with Artificial Intelligence: Theory and Applications. Cham pp. 251-276. Springer Nature SwitzerlandAbstract: In economics and many other forecasting domains, the real world problems are too complex for a single model that assumes a specific data generation process. The forecasting performance of different methods changesChange(s) depending on the nature of the time series. When forecasting large collections of time series, two lines of approaches have been developed using time series features, namely feature-based model selection and feature-based model combination. This chapter discusses the state-of-the-art feature-based methods, with reference to open-source software implementationsImplementation.BibTeX:
@incollection{LiL2023ForecastingLarge, author = {Li, Li and Li, Feng and Kang, Yanfei}, editor = {Hamoudia, Mohsen and Makridakis, Spyros and Spiliotis, Evangelos}, title = {Forecasting Large Collections of Time Series: Feature-Based Methods}, booktitle = {Forecasting with Artificial Intelligence: Theory and Applications}, publisher = {Springer Nature Switzerland}, year = {2023}, pages = {251--276}, url = {http://arxiv.org/abs/2309.13807}, doi = {10.1007/978-3-031-35879-1_10} }
-
Rui Pan, Tunan Ren, Baishan Guo, Feng Li, Guodong Li and Hansheng Wang (2022). “A Note on Distributed Quantile Regression by Pilot Sampling and One-Step Updating”. Journal of Business & Economic Statistics, Vol. 40(4), pp. 1691-1700.Abstract: Quantile regression is a method of fundamental importance. How to efficiently conduct quantile regression for a large dataset on a distributed system is of great importance. We show that the popularly used one-shot estimation is statistically inefficient if data are not randomly distributed across different workers. To fix the problem, a novel one-step estimation method is developed with the following nice properties. First, the algorithm is communication efficient. That is the communication cost demanded is practically acceptable. Second, the resulting estimator is statistically efficient. That is its asymptotic covariance is the same as that of the global estimator. Third, the estimator is robust against data distribution. That is its consistency is guaranteed even if data are not randomly distributed across different workers. Numerical experiments are provided to corroborate our findings. A real example is also presented for illustration.BibTeX:
@article{PanR2022NoteDistributed, author = {Pan, Rui and Ren, Tunan and Guo, Baishan and Li, Feng and Li, Guodong and Wang, Hansheng}, title = {A Note on Distributed Quantile Regression by Pilot Sampling and One-Step Updating}, journal = {Journal of Business & Economic Statistics}, year = {2022}, volume = {40}, number = {4}, pages = {1691--1700}, url = {https://www.researchgate.net/publication/354770486}, doi = {10.1080/07350015.2021.1961789} }
-
Xiaoqian Wang, Yanfei Kang, Fotios Petropoulos and Feng Li (2022). “The Uncertainty Estimation of Feature-Based Forecast Combinations”. Journal of the Operational Research Society, Vol. 73(5), pp. 979-993.Abstract: Forecasting is an indispensable element of operational research (OR) and an important aid to planning. The accurate estimation of the forecast uncertainty facilitates several operations management activities, predominantly in supporting decisions in inventory and supply chain management and effectively setting safety stocks. In this paper, we introduce a feature-based framework, which links the relationship between time series features and the interval forecasting performance into providing reliable interval forecasts. We propose an optimal threshold ratio searching algorithm and a new weight determination mechanism for selecting an appropriate subset of models and assigning combination weights for each time series tailored to the observed features. We evaluate our approach using a large set of time series from the M4 competition. Our experiments show that our approach significantly outperforms a wide range of benchmark models, both in terms of point forecasts as well as prediction intervals.BibTeX:
@article{WangX2022UncertaintyEstimation, author = {Wang, Xiaoqian and Kang, Yanfei and Petropoulos, Fotios and Li, Feng}, title = {The Uncertainty Estimation of Feature-Based Forecast Combinations}, journal = {Journal of the Operational Research Society}, year = {2022}, volume = {73}, number = {5}, pages = {979--993}, url = {https://arxiv.org/abs/1908.02891}, doi = {10.1080/01605682.2021.1880297} }
-
Zhiru Wang, Yu Pang, Mingxin Gan, Martin Skitmore and Feng Li (2022). “Escalator Accident Mechanism Analysis and Injury Prediction Approaches in Heavy Capacity Metro Rail Transit Stations”. Safety Science, Vol. 154pp. 105850.Abstract: The semi-open character with high passenger flow in Metro Rail Transport Stations (MRTS) makes safety management of human-electromechanical interaction escalator systems more complex. Safety management should not consider only single failures, but also the complex interactions in the system. This study applies task driven behavior theory and system theory to reveal a generic framework of the MRTS escalator accident mechanism and uses Lasso-Logistic Regression (LLR) for escalator injury prediction. Escalator accidents in the Beijing MRTS are used as a case study to estimate the applicability of the methodologies. The main results affirm that the application of System-Theoretical Process Analysis (STPA) and Task Driven Accident Process Analysis (TDAPA) to the generic escalator accident mechanism reveals non-failure state task driven passenger behaviors and constraints on safety that are not addressed in previous studies. The results also confirm that LLR is able to predict escalator accidents where there is a relatively large number of variables with limited observations. Additionally, increasing the amount of data improves the prediction accuracy for all three types of injuries in the case study, suggesting the LLR model has good extrapolation ability. The results can be applied in MRTS as instruments for both escalator accident investigation and accident prevention.BibTeX:
@article{WangZ2022EscalatorAccident, author = {Wang, Zhiru and Pang, Yu and Gan, Mingxin and Skitmore, Martin and Li, Feng}, title = {Escalator Accident Mechanism Analysis and Injury Prediction Approaches in Heavy Capacity Metro Rail Transit Stations}, journal = {Safety Science}, year = {2022}, volume = {154}, pages = {105850}, doi = {10.1016/j.ssci.2022.105850} }
-
Matthias Anderer and Feng Li (2022). “Hierarchical Forecasting with a Top-down Alignment of Independent-Level Forecasts”. International Journal of Forecasting, Vol. 38(4), pp. 1405-1414.Abstract: Hierarchical forecasting with intermittent time series is a challenge in both research and empirical studies. Extensive research focuses on improving the accuracy of each hierarchy, especially the intermittent time series at bottom levels. Then, hierarchical reconciliation can be used to improve the overall performance further. In this paper, we present a hierarchical-forecasting-with-alignment approach that treats the bottom-level forecasts as mutable to ensure higher forecasting accuracy on the upper levels of the hierarchy. We employ a pure deep learning forecasting approach, N-BEATS, for continuous time series at the top levels, and a widely used tree-based algorithm, LightGBM, for intermittent time series at the bottom level. The hierarchical-forecasting-with-alignment approach is a simple yet effective variant of the bottom-up method, accounting for biases that are difficult to observe at the bottom level. It allows suboptimal forecasts at the lower level to retain a higher overall performance. The approach in this empirical study was developed by the first author during the M5 Accuracy competition, ranking second place. The method is also business orientated and can be used to facilitate strategic business planning.BibTeX:
@article{AndererM2022HierarchicalForecasting, author = {Anderer, Matthias and Li, Feng}, title = {Hierarchical Forecasting with a Top-down Alignment of Independent-Level Forecasts}, journal = {International Journal of Forecasting}, year = {2022}, volume = {38}, number = {4}, pages = {1405--1414}, url = {https://arxiv.org/abs/2103.08250}, doi = {10.1016/j.ijforecast.2021.12.015} }
-
Fotios Petropoulos, Daniele Apiletti, Vassilios Assimakopoulos, Mohamed Zied Babai, Devon K. Barrow, Souhaib Ben Taieb, Christoph Bergmeir, Ricardo J. Bessa, Jakub Bijak, John E. Boylan, Jethro Browell, Claudio Carnevale, Jennifer L. Castle, Pasquale Cirillo, Michael P. Clements, Clara Cordeiro, Fernando Luiz Cyrino Oliveira, Shari De Baets, Alexander Dokumentov, Joanne Ellison, Piotr Fiszeder, Philip Hans Franses, David T. Frazier, Michael Gilliland, M. Sinan Gönül, Paul Goodwin, Luigi Grossi, Yael Grushka-Cockayne, Mariangela Guidolin, Massimo Guidolin, Ulrich Gunter, Xiaojia Guo, Renato Guseo, Nigel Harvey, David F. Hendry, Ross Hollyman, Tim Januschowski, Jooyoung Jeon, Victor Richmond R. Jose, Yanfei Kang, Anne B. Koehler, Stephan Kolassa, Nikolaos Kourentzes, Sonia Leva, Feng Li, Konstantia Litsiou, Spyros Makridakis, Gael M. Martin, Andrew B. Martinez, Sheik Meeran, Theodore Modis, Konstantinos Nikolopoulos, Dilek Önkal, Alessia Paccagnini, Anastasios Panagiotelis, Ioannis Panapakidis, Jose M. Pavía, Manuela Pedio, Diego J. Pedregal, Pierre Pinson, Patrícia Ramos, David E. Rapach, J. James Reade, Bahman Rostami-Tabar, Michał Rubaszek, Georgios Sermpinis, Han Lin Shang, Evangelos Spiliotis, Aris A. Syntetos, Priyanga Dilini Talagala, Thiyanga S. Talagala, Len Tashman, Dimitrios Thomakos, Thordis Thorarinsdottir, Ezio Todini, Juan Ramón Trapero Arenas, Xiaoqian Wang, Robert L. Winkler, Alisa Yusupova and Florian Ziel (2022). “Forecasting: Theory and Practice”. International Journal of Forecasting, Vol. 38(3), pp. 705-871.Abstract: Forecasting has always been at the forefront of decision making and planning. The uncertainty that surrounds the future is both exciting and challenging, with individuals and organisations seeking to minimise risks and maximise utilities. The large number of forecasting applications calls for a diverse set of forecasting methods to tackle real-life challenges. This article provides a non-systematic review of the theory and the practice of forecasting. We provide an overview of a wide range of theoretical, state-of-the-art models, methods, principles, and approaches to prepare, produce, organise, and evaluate forecasts. We then demonstrate how such theoretical concepts are applied in a variety of real-life contexts. We do not claim that this review is an exhaustive list of methods and applications. However, we wish that our encyclopedic presentation will offer a point of reference for the rich work that has been undertaken over the last decades, with some key insights for the future of forecasting theory and practice. Given its encyclopedic nature, the intended mode of reading is non-linear. We offer cross-references to allow the readers to navigate through the various topics. We complement the theoretical concepts and applications covered by large lists of free or open-source software implementations and publicly-available databases.BibTeX:
@article{PetropoulosF2022ForecastingTheory, author = {Petropoulos, Fotios and Apiletti, Daniele and Assimakopoulos, Vassilios and Babai, Mohamed Zied and Barrow, Devon K. and Ben Taieb, Souhaib and Bergmeir, Christoph and Bessa, Ricardo J. and Bijak, Jakub and Boylan, John E. and Browell, Jethro and Carnevale, Claudio and Castle, Jennifer L. and Cirillo, Pasquale and Clements, Michael P. and Cordeiro, Clara and Cyrino Oliveira, Fernando Luiz and De Baets, Shari and Dokumentov, Alexander and Ellison, Joanne and Fiszeder, Piotr and Franses, Philip Hans and Frazier, David T. and Gilliland, Michael and Gönül, M. Sinan and Goodwin, Paul and Grossi, Luigi and Grushka-Cockayne, Yael and Guidolin, Mariangela and Guidolin, Massimo and Gunter, Ulrich and Guo, Xiaojia and Guseo, Renato and Harvey, Nigel and Hendry, David F. and Hollyman, Ross and Januschowski, Tim and Jeon, Jooyoung and Jose, Victor Richmond R. and Kang, Yanfei and Koehler, Anne B. and Kolassa, Stephan and Kourentzes, Nikolaos and Leva, Sonia and Li, Feng and Litsiou, Konstantia and Makridakis, Spyros and Martin, Gael M. and Martinez, Andrew B. and Meeran, Sheik and Modis, Theodore and Nikolopoulos, Konstantinos and Önkal, Dilek and Paccagnini, Alessia and Panagiotelis, Anastasios and Panapakidis, Ioannis and Pavía, Jose M. and Pedio, Manuela and Pedregal, Diego J. and Pinson, Pierre and Ramos, Patrícia and Rapach, David E. and Reade, J. James and Rostami-Tabar, Bahman and Rubaszek, Michał and Sermpinis, Georgios and Shang, Han Lin and Spiliotis, Evangelos and Syntetos, Aris A. and Talagala, Priyanga Dilini and Talagala, Thiyanga S. and Tashman, Len and Thomakos, Dimitrios and Thorarinsdottir, Thordis and Todini, Ezio and Trapero Arenas, Juan Ramón and Wang, Xiaoqian and Winkler, Robert L. and Yusupova, Alisa and Ziel, Florian}, title = {Forecasting: Theory and Practice}, journal = {International Journal of Forecasting}, year = {2022}, volume = {38}, number = {3}, pages = {705--871}, url = {https://arxiv.org/abs/2012.03854}, doi = {10.1016/j.ijforecast.2021.11.001} }
-
Thiyanga S. Talagala, Feng Li and Yanfei Kang (2022). “FFORMPP: Feature-Based Forecast Model Performance Prediction”. International Journal of Forecasting, Vol. 38(3), pp. 920-943.Abstract: This paper introduces a novel meta-learning algorithm for time series forecast model performance prediction. We model the forecast error as a function of time series features calculated from historical time series with an efficient Bayesian multivariate surface regression approach. The minimum predicted forecast error is then used to identify an individual model or a combination of models to produce the final forecasts. It is well known that the performance of most meta-learning models depends on the representativeness of the reference dataset used for training. In such circumstances, we augment the reference dataset with a feature-based time series simulation approach, namely GRATIS, to generate a rich and representative time series collection. The proposed framework is tested using the M4 competition data and is compared against commonly used forecasting approaches. Our approach provides comparable performance to other model selection and combination approaches but at a lower computational cost and a higher degree of interpretability, which is important for supporting decisions. We also provide useful insights regarding which forecasting models are expected to work better for particular types of time series, the intrinsic mechanisms of the meta-learners, and how the forecasting performance is affected by various factors.BibTeX:
@article{TalagalaTS2022FFORMPPFeaturebased, author = {Talagala, Thiyanga S. and Li, Feng and Kang, Yanfei}, title = {FFORMPP: Feature-Based Forecast Model Performance Prediction}, journal = {International Journal of Forecasting}, year = {2022}, volume = {38}, number = {3}, pages = {920--943}, url = {https://arxiv.org/abs/1908.11500}, doi = {10.1016/j.ijforecast.2021.07.002} }
-
Yanfei Kang, Wei Cao, Fotios Petropoulos and Feng Li (2022). “Forecast with Forecasts: Diversity Matters”. European Journal of Operational Research, Vol. 301(1), pp. 180-190.Abstract: Forecast combinations have been widely applied in the last few decades to improve forecasting. Estimating optimal weights that can outperform simple averages is not always an easy task. In recent years, the idea of using time series features for forecast combinations has flourished. Although this idea has been proved to be beneficial in several forecasting competitions, it may not be practical in many situations. For example, the task of selecting appropriate features to build forecasting models is often challenging. Even if there was an acceptable way to define the features, existing features are estimated based on the historical patterns, which are likely to change in the future. Other times, the estimation of the features is infeasible due to limited historical data. In this work, we suggest a change of focus from the historical data to the produced forecasts to extract features. We use out-of-sample forecasts to obtain weights for forecast combinations by amplifying the diversity of the pool of methods being combined. A rich set of time series is used to evaluate the performance of the proposed method. Experimental results show that our diversity-based forecast combination framework not only simplifies the modeling process but also achieves superior forecasting performance in terms of both point forecasts and prediction intervals. The value of our proposition lies on its simplicity, transparency, and computational efficiency, elements that are important from both an optimization and a decision analysis perspective.BibTeX:
@article{KangY2022ForecastForecasts, author = {Kang, Yanfei and Cao, Wei and Petropoulos, Fotios and Li, Feng}, title = {Forecast with Forecasts: Diversity Matters}, journal = {European Journal of Operational Research}, year = {2022}, volume = {301}, number = {1}, pages = {180--190}, url = {https://arxiv.org/abs/2012.01643}, doi = {10.1016/j.ejor.2021.10.024} }
-
Xuening Zhu, Feng Li and Hansheng Wang (2021). “Least-Square Approximation for a Distributed System”. Journal of Computational and Graphical Statistics, Vol. 30(4), pp. 1004-1018.Abstract: In this work, we develop a distributed least-square approximation (DLSA) method that is able to solve a large family of regression problems (e.g., linear regression, logistic regression, and Cox’s model) on a distributed system. By approximating the local objective function using a local quadratic form, we are able to obtain a combined estimator by taking a weighted average of local estimators. The resulting estimator is proved to be statistically as efficient as the global estimator. Moreover, it requires only one round of communication. We further conduct a shrinkage estimation based on the DLSA estimation using an adaptive Lasso approach. The solution can be easily obtained by using the LARS algorithm on the master node. It is theoretically shown that the resulting estimator possesses the oracle property and is selection consistent by using a newly designed distributed Bayesian information criterion. The finite sample performance and computational efficiency are further illustrated by an extensive numerical study and an airline dataset. The airline dataset is 52 GB in size. The entire methodology has been implemented in Python for a de-facto standard Spark system. The proposed DLSA algorithm on the Spark system takes 26 min to obtain a logistic regression estimator, which is more efficient and memory friendly than conventional methods. Supplementary materials for this article are available online.BibTeX:
@article{ZhuX2021LeastSquareApproximation, author = {Zhu, Xuening and Li, Feng and Wang, Hansheng}, title = {Least-Square Approximation for a Distributed System}, journal = {Journal of Computational and Graphical Statistics}, year = {2021}, volume = {30}, number = {4}, pages = {1004--1018}, url = {https://arxiv.org/abs/1908.04904}, doi = {10.1080/10618600.2021.1923517} }
-
Yanfei Kang, Evangelos Spiliotis, Fotios Petropoulos, Nikolaos Athiniotis, Feng Li and Vassilios Assimakopoulos (2021). “Déjà vu: A Data-Centric Forecasting Approach through Time Series Cross-Similarity”. Journal of Business Research, Vol. 132pp. 719-731.Abstract: Accurate forecasts are vital for supporting the decisions of modern companies. Forecasters typically select the most appropriate statistical model for each time series. However, statistical models usually presume some data generation process while making strong assumptions about the errors. In this paper, we present a novel data-centric approach — ‘forecasting with cross-similarity’, which tackles model uncertainty in a model-free manner. Existing similarity-based methods focus on identifying similar patterns within the series, i.e., ‘self-similarity’. In contrast, we propose searching for similar patterns from a reference set, i.e., ‘cross-similarity’. Instead of extrapolating, the future paths of the similar series are aggregated to obtain the forecasts of the target series. Building on the cross-learning concept, our approach allows the application of similarity-based forecasting on series with limited lengths. We evaluate the approach using a rich collection of real data and show that it yields competitive accuracy in both points forecasts and prediction intervals.BibTeX:
@article{KangY2021DejaVu, author = {Kang, Yanfei and Spiliotis, Evangelos and Petropoulos, Fotios and Athiniotis, Nikolaos and Li, Feng and Assimakopoulos, Vassilios}, title = {Déjà vu: A Data-Centric Forecasting Approach through Time Series Cross-Similarity}, journal = {Journal of Business Research}, year = {2021}, volume = {132}, pages = {719--731}, url = {https://arxiv.org/abs/1909.00221}, doi = {10.1016/j.jbusres.2020.10.051} }
-
Megan G. Janeway, Xiang Zhao, Max Rosenthaler, Yi Zuo, Kumar Balasubramaniyan, Michael Poulson, Miriam Neufeld, Jeffrey J. Siracuse, Courtney E. Takahashi, Lisa Allee, Tracey Dechert, Peter A. Burke, Feng Li and Bindu Kalesan (2021). “Clinical Diagnostic Phenotypes in Hospitalizations Due to Self-Inflicted Firearm Injury”. Journal of Affective Disorders, Vol. 278pp. 172-180.Abstract: Hospitalized self-inflicted firearm injuries have not been extensively studied, particularly regarding clinical diagnoses at the index admission. The objective of this study was to discover the diagnostic phenotypes (DPs) or clusters of hospitalized self-inflicted firearm injuries. Using Nationwide Inpatient Sample data in the US from 1993 to 2014, we used International Classification of Diseases, Ninth Revision codes to identify self-inflicted firearm injuries among those ≥18 years of age. The 25 most frequent diagnostic codes were used to compute a dissimilarity matrix and the optimal number of clusters. We used hierarchical clustering to identify the main DPs. The overall cohort included 14072 hospitalizations, with self-inflicted firearm injuries occurring mainly in those between 16 to 45 years of age, black, with co-occurring tobacco and alcohol use, and mental illness. Out of the three identified DPs, DP1 was the largest (n=10,110), and included most common diagnoses similar to overall cohort, including major depressive disorders (27.7%), hypertension (16.8%), acute post hemorrhagic anemia (16.7%), tobacco (15.7%) and alcohol use (12.6%). DP2 (n=3,725) was not characterized by any of the top 25 ICD-9 diagnoses codes, and included children and peripartum women. DP3, the smallest phenotype (n=237), had high prevalence of depression similar to DP1, and defined by fewer fatal injuries of chest and abdomen. There were three distinct diagnostic phenotypes in hospitalizations due to self-inflicted firearm injuries. Further research is needed to determine how DPs can be used to tailor clinical care and prevention efforts.BibTeX:
@article{JanewayMG2021ClinicalDiagnostic, author = {Janeway, Megan G. and Zhao, Xiang and Rosenthaler, Max and Zuo, Yi and Balasubramaniyan, Kumar and Poulson, Michael and Neufeld, Miriam and Siracuse, Jeffrey J. and Takahashi, Courtney E. and Allee, Lisa and Dechert, Tracey and Burke, Peter A. and Li, Feng and Kalesan, Bindu}, title = {Clinical Diagnostic Phenotypes in Hospitalizations Due to Self-Inflicted Firearm Injury}, journal = {Journal of Affective Disorders}, year = {2021}, volume = {278}, pages = {172--180}, doi = {10.1016/j.jad.2020.09.067} }
-
Bindu Kalesan, Siran Zhao, Michael Poulson, Miriam Neufeld, Tracey Dechert, Jeffrey J. Siracuse, Yi Zuo and Feng Li (2020). “Intersections of Firearm Suicide, Drug-Related Mortality, and Economic Dependency in Rural America”. Journal of Surgical Research, Vol. 256pp. 96-102. ElsevierBibTeX:
@article{KalesanB2020IntersectionsFirearm, author = {Kalesan, Bindu and Zhao, Siran and Poulson, Michael and Neufeld, Miriam and Dechert, Tracey and Siracuse, Jeffrey J and Zuo, Yi and Li, Feng}, title = {Intersections of Firearm Suicide, Drug-Related Mortality, and Economic Dependency in Rural America}, journal = {Journal of Surgical Research}, publisher = {Elsevier}, year = {2020}, volume = {256}, pages = {96--102}, doi = {10.1016/j.jss.2020.06.011} }
-
Xixi Li, Yanfei Kang and Feng Li (2020). “Forecasting with Time Series Imaging”. Expert Systems with Applications, Vol. 160pp. 113680.Abstract: Feature-based time series representations have attracted substantial attention in a wide range of time series analysis methods. Recently, the use of time series features for forecast model averaging has been an emerging research focus in the forecasting community. Nonetheless, most of the existing approaches depend on the manual choice of an appropriate set of features. Exploiting machine learning methods to extract features from time series automatically becomes crucial in state-of-the-art time series analysis. In this paper, we introduce an automated approach to extract time series features based on time series imaging. We first transform time series into recurrence plots, from which local features can be extracted using computer vision algorithms. The extracted features are used for forecast model averaging. Our experiments show that forecasting based on automatically extracted features, with less human intervention and a more comprehensive view of the raw time series data, yields highly comparable performances with the best methods in the largest forecasting competition dataset (M4) and outperforms the top methods in the Tourism forecasting competition dataset.BibTeX:
@article{LiX2020ForecastingTime, author = {Li, Xixi and Kang, Yanfei and Li, Feng}, title = {Forecasting with Time Series Imaging}, journal = {Expert Systems with Applications}, year = {2020}, volume = {160}, pages = {113680}, url = {https://arxiv.org/abs/1904.08064}, doi = {10.1016/j.eswa.2020.113680} }
-
Chengcheng Hao, Feng Li and Dietrich von Rosen (2020). “A Bilinear Reduced Rank Model”. In Contemporary Experimental Design, Multivariate Analysis and Data Mining. Springer NatureAbstract: This article considers a bilinear model that includes two different latent effects. The first effect has a direct influence on the response variable, whereas the second latent effect is assumed to first influence other latent variables, which in turn affect the response variable. In this article, latent variables are modelled via rank restrictions on unknown mean parameters and the models which are used are often referred to as reduced rank regression models. This article presents a likelihood-based approach that results in explicit estimators. In our model, the latent variables act as covariates that we know exist, but their direct influence is unknown and will therefore not be considered in detail. One example is if we observe hundreds of weather variables, but we cannot say which or how these variables affect plant growth.BibTeX:
@incollection{HaoC2020BilinearReduced, author = {Hao, Chengcheng and Li, Feng and von Rosen, Dietrich}, editor = {Fan, Jianqing and Pan, Jianxin}, title = {A Bilinear Reduced Rank Model}, booktitle = {Contemporary Experimental Design, Multivariate Analysis and Data Mining}, publisher = {Springer Nature}, year = {2020}, url = {https://www.researchgate.net/publication/341587390}, doi = {10.1007/978-3-030-46161-4_21} }
-
Yanfei Kang, Rob J. Hyndman and Feng Li (2020). “GRATIS: GeneRAting TIme Series with Diverse and Controllable Characteristics”. Statistical Analysis and Data Mining: The ASA Data Science Journal, Vol. 13(4), pp. 354-376.Abstract: The explosion of time series data in recent years has brought a flourish of new time series analysis methods, for forecasting, clustering, classification and other tasks. The evaluation of these new methods requires either collecting or simulating a diverse set of time series benchmarking data to enable reliable comparisons against alternative approaches. We propose GeneRAting TIme Series with diverse and controllable characteristics, named GRATIS, with the use of mixture autoregressive (MAR) models. We simulate sets of time series using MAR models and investigate the diversity and coverage of the generated time series in a time series feature space. By tuning the parameters of the MAR models, GRATIS is also able to efficiently generate new time series with controllable features. In general, as a costless surrogate to the traditional data collection approach, GRATIS can be used as an evaluation tool for tasks such as time series forecasting and classification. We illustrate the usefulness of our time series generation process through a time series forecasting application.BibTeX:
@article{KangY2020GRATISGeneRAting, author = {Kang, Yanfei and Hyndman, Rob J. and Li, Feng}, title = {GRATIS: GeneRAting TIme Series with Diverse and Controllable Characteristics}, journal = {Statistical Analysis and Data Mining: The ASA Data Science Journal}, year = {2020}, volume = {13}, number = {4}, pages = {354--376}, url = {https://arxiv.org/abs/1903.02787}, doi = {10.1002/sam.11461} }
-
BibTeX:
@book{kang2020fppcn, author = {康雁飞 and 李丰}, title = {预测:方法与实践}, publisher = {在线出版}, year = {2020}, url = {https://otexts.com/fppcn/} }
-
BibTeX:
@book{kang2020statcompcn, author = {康雁飞 and 李丰}, title = {统计计算}, publisher = {在线出版}, year = {2020}, url = {https://feng.li/files/statscompbook/} }
-
Hannah M. Bailey, Yi Zuo, Feng Li, Jae Min, Krishna Vaddiparti, Mattia Prosperi, Jeffrey Fagan, Sandro Galea and Bindu Kalesan (2019). “Changes in Patterns of Mortality Rates and Years of Life Lost Due to Firearms in the United States, 1999 to 2016: A Joinpoint Analysis”. PLOS ONE, Vol. 14(11), pp. e0225223. Public Library of ScienceAbstract: Background Firearm-related death rates and years of potential life lost (YPLL) vary widely between population subgroups and states. However, changes or inflections in temporal trends within subgroups and states are not fully documented. We assessed temporal patterns and inflections in the rates of firearm deaths and %YPLL due to firearms for overall and by sex, age, race/ethnicity, intent, and states in the United States between 1999 and 2016. Methods We extracted age-adjusted firearm mortality and YPLL rates per 100,000, and %YPLL from 1999 to 2016 by using the WONDER (Wide-ranging Online Data for Epidemiologic Research) database. We used Joinpoint Regression to assess temporal trends, the inflection points, and annual percentage change (APC) from 1999 to 2016. Results National firearm mortality rates were 10.3 and 11.8 per 100,000 in 1999 and 2016, with two distinct segments; a plateau until 2014 followed by an increase of APC = 7.2% (95% CI 3.1, 11.4). YPLL rates were from 304.7 and 338.2 in 1999 and 2016 with a steady APC increase in %YPLL of 0.65% (95% CI 0.43, 0.87) from 1999 to an inflection point in 2014, followed by a larger APC in %YPLL of 5.1% (95% CI 0.1, 10.4). The upward trend in firearm mortality and YPLL rates starting in 2014 was observed in subgroups of male, non-Hispanic blacks, Hispanic whites and for firearm assaults. The inflection points for firearm mortality and YPLL rates also varied across states. Conclusions Within the United States, firearm mortality rates and YPLL remained constant between 1999 and 2014 and has been increasing subsequently. There was, however, an increase in firearm mortality rates in several subgroups and individual states earlier than 2014.BibTeX:
@article{BaileyHM2019ChangesPatterns, author = {Bailey, Hannah M. and Zuo, Yi and Li, Feng and Min, Jae and Vaddiparti, Krishna and Prosperi, Mattia and Fagan, Jeffrey and Galea, Sandro and Kalesan, Bindu}, title = {Changes in Patterns of Mortality Rates and Years of Life Lost Due to Firearms in the United States, 1999 to 2016: A Joinpoint Analysis}, journal = {PLOS ONE}, publisher = {Public Library of Science}, year = {2019}, volume = {14}, number = {11}, pages = {e0225223}, doi = {10.1371/journal.pone.0225223} }
-
Feng Li and Zhuojing He (2019). “Credit Risk Clustering in a Business Group: Which Matters More, Systematic or Idiosyncratic Risk?”. Cogent Economics & Finance, Vol. 7(1), pp. 1632528.Abstract: Understanding how defaults correlate across firms is a persistent concern in risk management. In this paper, we apply covariate-dependent copula models to assess the dynamic nature of credit risk dependence, which we define as “credit risk clustering”. We also study the driving forces of the credit risk clustering in CEC business group in China. Our empirical analysis shows that the credit risk clustering varies over time and exhibits different patterns across firm pairs in a business group. We also investigate the impacts of systematic and idiosyncratic factors on credit risk clustering. We find that the impacts of the money supply and the short-term interest rates are positive, whereas the impacts of exchange rates are negative. The roles of the CPI on credit risk clustering are ambiguous. Idiosyncratic factors are vital for predicting credit risk clustering. From a policy perspective, our results not only strengthen the results of previous research but also provide a possible approach to model and predict the extreme co-movement of credit risk in business groups with financial indicators.BibTeX:
@article{LiF2019CreditRisk, author = {Li, Feng and He, Zhuojing}, editor = {McMillan, David}, title = {Credit Risk Clustering in a Business Group: Which Matters More, Systematic or Idiosyncratic Risk?}, journal = {Cogent Economics & Finance}, year = {2019}, volume = {7}, number = {1}, pages = {1632528}, url = {http://doi.org/10.2139/ssrn.3182925}, doi = {10.1080/23322039.2019.1632528} }
-
Elizabeth C. Pino, Yi Zuo, Camila Maciel De Olivera, Shruthi Mahalingaiah, Olivia Keiser, Lynn L. Moore, Feng Li, Ramachandran S. Vasan, Barbara E. Corkey and Bindu Kalesan (2018). “Cohort Profile: The MULTI sTUdy Diabetes rEsearch (MULTITUDE) Consortium”. BMJ Open, Vol. 8(5), pp. e020640.Abstract: Purpose Globally, the age-standardised prevalence of type 2 diabetes mellitus (T2DM) has nearly doubled from 1980 to 2014, rising from 4.7% to 8.5% with an estimated 422 million adults living with the chronic disease. The MULTI sTUdy Diabetes rEsearch (MULTITUDE) consortium was recently established to harmonise data from 17 independent cohort studies and clinical trials and to facilitate a better understanding of the determinants, risk factors and outcomes associated with T2DM. Participants Participants range in age from 3 to 88 years at baseline, including both individuals with and without T2DM. MULTITUDE is an individual-level pooled database of demographics, comorbidities, relevant medications, clinical laboratory values, cardiac health measures, and T2DM-associated events and outcomes across 45 US states and the District of Columbia. Findings to date Among the 135 156 ongoing participants included in the consortium, almost 25% (33 421) were diagnosed with T2DM at baseline. The average age of the participants was 54.3, while the average age of participants with diabetes was 64.2. Men (55.3%) and women (44.6%) were almost equally represented across the consortium. Non-whites accounted for 31.6% of the total participants and 40% of those diagnosed with T2DM. Fewer individuals with diabetes reported being regular smokers than their non-diabetic counterparts (40.3% vs 47.4%). Over 85% of those with diabetes were reported as either overweight or obese at baseline, compared with 60.7% of those without T2DM. We observed differences in all-cause mortality, overall and by T2DM status, between cohorts. Future plans Given the wide variation in demographics and all-cause mortality in the cohorts, MULTITUDE consortium will be a unique resource for conducting research to determine: differences in the incidence and progression of T2DM; sequence of events or biomarkers prior to T2DM diagnosis; disease progression from T2DM to disease-related outcomes, complications and premature mortality; and to assess race/ethnicity differences in the above associations.BibTeX:
@article{PinoEC2018CohortProfile, author = {Pino, Elizabeth C. and Zuo, Yi and Olivera, Camila Maciel De and Mahalingaiah, Shruthi and Keiser, Olivia and Moore, Lynn L. and Li, Feng and Vasan, Ramachandran S. and Corkey, Barbara E. and Kalesan, Bindu}, title = {Cohort Profile: The MULTI sTUdy Diabetes rEsearch (MULTITUDE) Consortium}, journal = {BMJ Open}, year = {2018}, volume = {8}, number = {5}, pages = {e020640}, doi = {10.1136/bmjopen-2017-020640} }
-
Feng Li and Yanfei Kang (2018). “Improving Forecasting Performance Using Covariate-Dependent Copula Models”. International Journal of Forecasting, Vol. 34(3), pp. 456-476.Abstract: Copulas provide an attractive approach to the construction of multivariate distributions with flexible marginal distributions and different forms of dependences. Of particular importance in many areas is the possibility of forecasting the tail-dependences explicitly. Most of the available approaches are only able to estimate tail-dependences and correlations via nuisance parameters, and cannot be used for either interpretation or forecasting. We propose a general Bayesian approach for modeling and forecasting tail-dependences and correlations as explicit functions of covariates, with the aim of improving the copula forecasting performance. The proposed covariate-dependent copula model also allows for Bayesian variable selection from among the covariates of the marginal models, as well as the copula density. The copulas that we study include the Joe-Clayton copula, the Clayton copula, the Gumbel copula and the Student’s t-copula. Posterior inference is carried out using an efficient MCMC simulation method. Our approach is applied to both simulated data and the S&P 100 and S&P 600 stock indices. The forecasting performance of the proposed approach is compared with those of other modeling strategies based on log predictive scores. A value-at-risk evaluation is also performed for the model comparisons.BibTeX:
@article{LiF2018ImprovingForecasting, author = {Li, Feng and Kang, Yanfei}, title = {Improving Forecasting Performance Using Covariate-Dependent Copula Models}, journal = {International Journal of Forecasting}, year = {2018}, volume = {34}, number = {3}, pages = {456--476}, url = {https://arxiv.org/abs/1401.0100}, doi = {10.1016/j.ijforecast.2018.01.007} }
-
BibTeX:
@book{li2016distributedcn, author = {李丰}, title = {大数据分布式计算与案例}, publisher = {中国人民大学出版社}, year = {2016}, edition = {第一版}, url = {https://feng.li/files/distcompbook/} }
-
Feng Li and Mattias Villani (2013). “Efficient Bayesian Multivariate Surface Regression”. Scandinavian Journal of Statistics, Vol. 40(4), pp. 706-723.Abstract: Methods for choosing a fixed set of knot locations in additive spline models are fairly well established in the statistical literature. The curse of dimensionality makes it nontrivial to extend these methods to nonadditive surface models, especially when there are more than a couple of covariates. We propose a multivariate Gaussian surface regression model that combines both additive splines and interactive splines, and a highly efficient Markov chain Monte Carlo algorithm that updates all the knot locations jointly. We use shrinkage prior to avoid overfitting with different estimated shrinkage factors for the additive and surface part of the model, and also different shrinkage parameters for the different response variables. Simulated data and an application to firm leverage data show that the approach is computationally efficient, and that allowing for freely estimated knot locations can offer a substantial improvement in out-of-sample predictive performance.BibTeX:
@article{LiF2013EfficientBayesian, author = {Li, Feng and Villani, Mattias}, title = {Efficient Bayesian Multivariate Surface Regression}, journal = {Scandinavian Journal of Statistics}, year = {2013}, volume = {40}, number = {4}, pages = {706--723}, url = {https://arxiv.org/abs/1110.3689}, doi = {10.1111/sjos.12022} }
-
Feng Li (2013). “Bayesian Modeling of Conditional Densities”. Thesis at: Department of Statistics, Stockholm University.Abstract: This thesis develops models and associated Bayesian inference methods for flexible univariate and multivariate conditional density estimation. The models are flexible in the sense that they can capture widely differing shapes of the data. The estimation methods are specifically designed to achieve flexibility while still avoiding overfitting. The models are flexible both for a given covariate value, but also across covariate space. A key contribution of this thesis is that it provides general approaches of density estimation with highly efficient Markov chain Monte Carlo methods. The methods are illustrated on several challenging non-linear and non-normal datasets. In the first paper, a general model is proposed for flexibly estimating the density of a continuous response variable conditional on a possibly high-dimensional set of covariates. The model is a finite mixture of asymmetric student-t densities with covariate-dependent mixture weights. The four parameters of the components, the mean, degrees of freedom, scale and skewness, are all modeled as functions of the covariates. The second paper explores how well a smooth mixture of symmetric components can capture skewed data. Simulations and applications on real data show that including covariate-dependent skewness in the components can lead to substantially improved performance on skewed data, often using a much smaller number of components. We also introduce smooth mixtures of gamma and log-normal components to model positively-valued response variables. In the third paper we propose a multivariate Gaussian surface regression model that combines both additive splines and interactive splines, and a highly efficient MCMC algorithm that updates all the multi-dimensional knot locations jointly. We use shrinkage priors to avoid overfitting with different estimated shrinkage factors for the additive and surface part of the model, and also different shrinkage parameters for the different response variables. In the last paper we present a general Bayesian approach for directly modeling dependencies between variables as function of explanatory variables in a flexible copula context. In particular, the Joe-Clayton copula is extended to have covariate-dependent tail dependence and correlations. Posterior inference is carried out using a novel and efficient simulation method. The appendix of the thesis documents the computational implementation details.BibTeX:
@thesis{LiF2013BayesianModeling, author = {Li, Feng}, title = {Bayesian Modeling of Conditional Densities}, school = {Department of Statistics, Stockholm University}, year = {2013}, url = {http://urn.kb.se/resolve?urn=urn:nbn:se:su:diva-89426} }
-
Feng Li, Mattias Villani and Robert Kohn (2011). “Modelling Conditional Densities Using Finite Smooth Mixtures”. In Mixtures: Estimation and Applications. pp. 123-144. John Wiley & SonsAbstract: Smooth mixtures, i.e. mixture models with covariate-dependent mixing weights, are very useful flexible models for conditional densities. Previous work shows that using too simple mixture components for modeling heteroscedastic and/or heavy tailed data can give a poor fit, even with a large number of components. This paper explores how well a smooth mixture of symmetric components can capture skewed data. Simulations and applications on real data show that including covariate-dependent skewness in the components can lead to substantially improved performance on skewed data, often using a much smaller number of components. Furthermore, variable selection is effective in removing unnecessary covariates in the skewness, which means that there is little loss in allowing for skewness in the components when the data are actually symmetric. We also introduce smooth mixtures of gamma and log-normal components to model positively-valued response variables.BibTeX:
@incollection{LiF2011ModellingConditional, author = {Li, Feng and Villani, Mattias and Kohn, Robert}, title = {Modelling Conditional Densities Using Finite Smooth Mixtures}, booktitle = {Mixtures: Estimation and Applications}, publisher = {John Wiley & Sons}, year = {2011}, pages = {123--144}, url = {https://archive.riksbank.se/en/Web-archive/Published/Other-reports/Working-Paper-Series/2010/No-245-Modeling-Conditional-Densities-Using-Finite-Smooth-Mixtures/index.html}, doi = {10.1002/9781119995678.ch6} }
-
Feng Li, Mattias Villani and Robert Kohn (2010). “Flexible Modeling of Conditional Distributions Using Smooth Mixtures of Asymmetric Student t Densities”. Journal of Statistical Planning and Inference, Vol. 140(12), pp. 3638-3654.Abstract: A general model is proposed for flexibly estimating the density of a continuous response variable conditional on a possibly high-dimensional set of covariates. The model is a finite mixture of asymmetric student t densities with covariate-dependent mixture weights. The four parameters of the components, the mean, degrees of freedom, scale and skewness, are all modeled as functions of the covariates. Inference is Bayesian and the computation is carried out using Markov chain Monte Carlo simulation. To enable model parsimony, a variable selection prior is used in each set of covariates and among the covariates in the mixing weights. The model is used to analyze the distribution of daily stock market returns, and shown to more accurately forecast the distribution of returns than other widely used models for financial data.BibTeX:
@article{LiF2010FlexibleModeling, author = {Li, Feng and Villani, Mattias and Kohn, Robert}, title = {Flexible Modeling of Conditional Distributions Using Smooth Mixtures of Asymmetric Student t Densities}, journal = {Journal of Statistical Planning and Inference}, year = {2010}, volume = {140}, number = {12}, pages = {3638--3654}, url = {https://archive.riksbank.se/en/Web-archive/Published/Other-reports/Working-Paper-Series/2009/No-233-Flexible-Modeling-of-Conditional-Distributions-Using-Smooth-Mixtures-of-Asymmetric-Student-T-Densities/index.html}, doi = {10.1016/j.jspi.2010.04.031} }